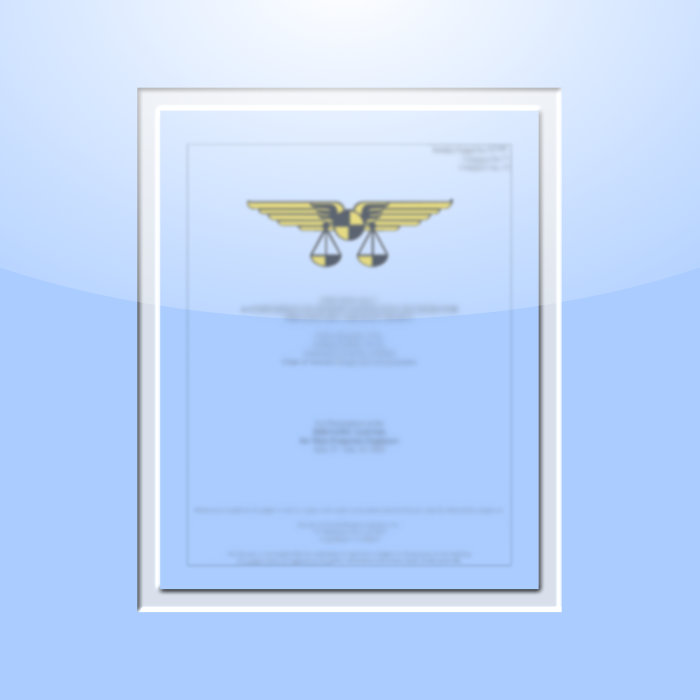
3579. An Empirical Aero Gas Turbine Preliminary Weight Estimation Method Based On Artificial Neural Networks
$20.00
SAWE Members get a $200 store credit each year.*
*Store credit coupon available at checkout, click the button in your shopping cart to apply the coupon.
Not applicable to SAWE textbooks and current conference technical papers.
Paper
Abstract
During the last years the number of parameters involved in the design and selection of an aero engine has greatly increased, making the decision for the most suitable aircraft engine at the preliminary design stage a challenging task. To cope with these requirements multi-disciplinary parametric tools, that perform Techno-economic and Environmental Risk Analysis (TERA) and trade-off studies have been introduced. These tools integrate several packages that model different aspects of the engine, aircraft and mission at the preliminary design stage. One of those is the preliminary weight estimation module, necessary for the aircraft performance, but also for the engine optimisation studies.
Existing aero gas turbine preliminary weight estimation methods that are publicly available either fail to achieve the necessary accuracy or are complex and time consuming. Moreover, these methods are based on engine databases that are more than 30 years old, rendering them outdated and untrustworthy for recent engines. Therefore, there is a need for a new, more accurate, simple and fast method.
The ability of empirical methods to better capture aspects that cannot be modelled easily, combined with the availability of data for the whole aero engine weight, led to the development of a new method based on an aero gas turbine database. Take-off thrust, overall pressure ratio, by-pass ratio and year of entry into service are the four key variables influencing the engine weight that were selected for the present study. To analyse the available data Artificial Neural Networks (ANNs) were selected as the most suitable tool, due to their ability to model effectively complex patterns and relations.
This paper includes an analysis of several possible feedforward backpropagation ANN configurations and their comparison based on accuracy, simplicity and calculation time with the two hidden layer emerging as the most suitable configuration. However, the error achieved is higher than the indicated limit of ~10% for engine optimisation studies. Therefore several improvements are suggested for expansion of the database and alternative configurations that will help reduce the calculated error.